Table of Contents
America’s large chain drug stores have been in a tough spot. Walgreens’ fourth quarter report shed light on a whopping $3.1 billion in net losses for the calendar year. Rite Aid filed for bankruptcy in October of last year, citing mounting debt and its ongoing legal battle over its role in the opiate crisis. CVS recently pledged to close 900 stores by the end of 2024.
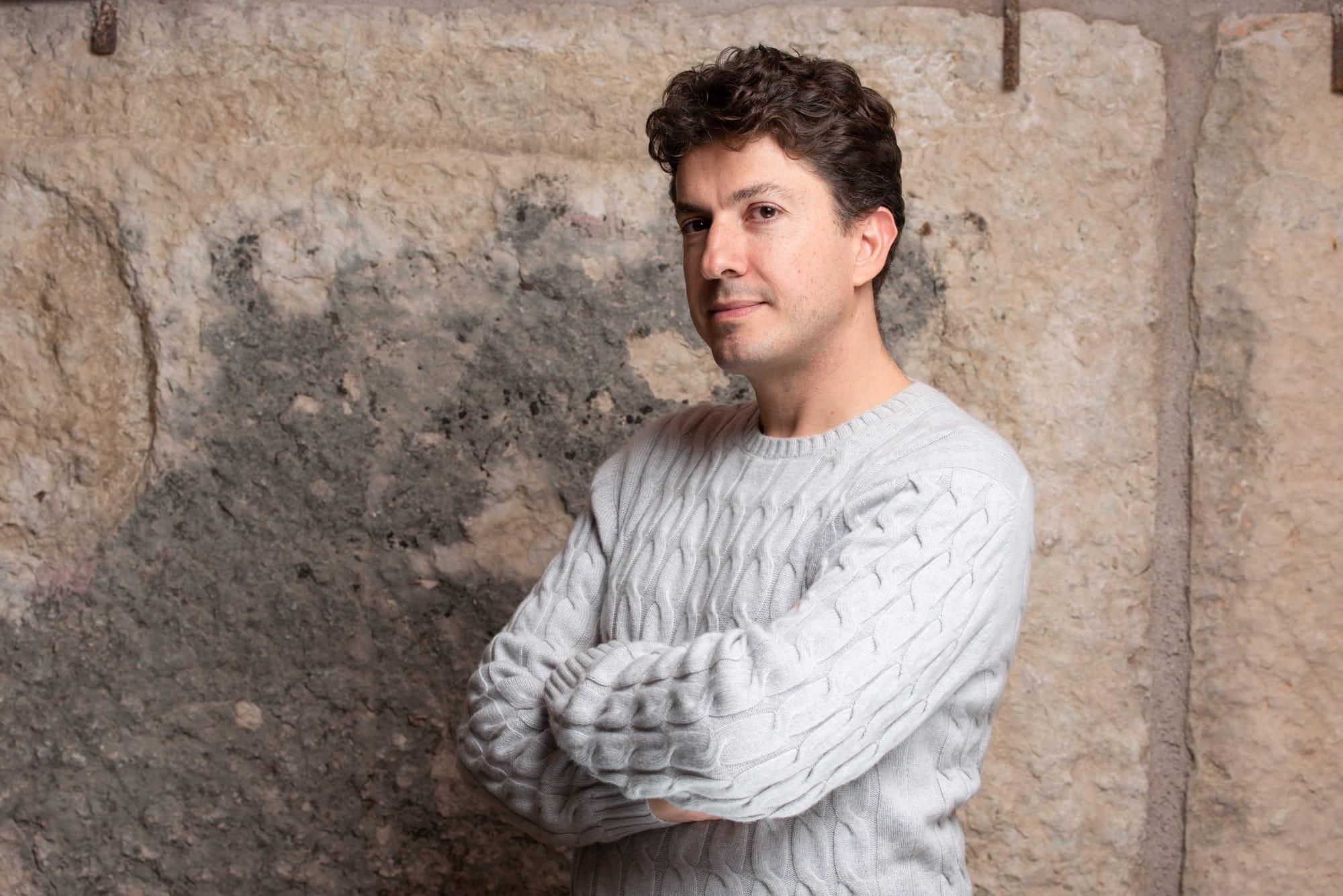
Fabrizio Fantini
Drug shortages, labor strikes, shoplifting and competition from online retailers have left executives of large chain pharmacies scrambling to restructure. In many cases, restructuring involves drastic cost-cutting measures such as layoffs, consolidation, or reducing a physical footprint and replacing it with online services. While these austerity measures are necessary, they limit potential for future growth. To ensure long-term success, these organizations shouldn’t just spend less, but spend more efficiently.
One inefficiency large chain pharmacies must tackle is their outdated approach to pricing. Suboptimal pricing strategies make even less sense when considering that large chain pharmacies have become one-stop-shops — 36% of customers buy food and grocery items, 32% buy beauty products, and 30% buy household items. If online pharmacies continue to gain traction in the coming decade, brick-and-mortar pharmacies should look to emphasize the “retail” in retail pharmacy, and that can only be done by freeing themselves from linear, inflexible pricing models.
A linear pricing model means the price of an item is directly proportional to the chance of a customer purchasing it. Traditional thinking dictates that the customers of large chain stores are highly price sensitive. If this were still the case, why have mass discounting efforts often been ineffective at remedying overstocks? The answer is because the price sensitivity among items at large chain drug stores is far from uniform — they house a mix of medium, high and very highly price sensitive products.
COVID and the ensuing supply chain crisis was a case study on the limits of linear pricing models. External factors such as public health, inflation and geopolitics guided the spending patterns of consumers, rather than price alone. Large chain pharmacies scarred by these global events may look to replace their outdated cost-plus pricing models with predictive models, but to do so is misguided. The problem with linear models isn’t that they can’t predict global pandemics or war — the problem is that they can’t respond fast enough. Dynamic, or value-based pricing models give large retail pharmacies the agility required to adapt to macroeconomic factors out of their control.
Shifting to a dynamic pricing model will not be an easy feat, as it requires honing-in on the right level of data granularity. Data granularity refers to the level of detail within a data set. If a pharmacy based its pricing solely off of yearly transactions across all stores nationwide, this data would have low granularity. On the other hand, high granularity would mean measuring an individual branch’s transactions by the second. In an industry where rapid replenishment is required, and a single stock-out can sever long-standing customer loyalties, a high level of data granularity is ideal.
However, a common mistake is overshooting the optimal level of data granularity — missing the forest for the trees. By using data that is too granular, AI forecasting models will incorrectly assume that they can predict stats such as daily sales per SKU, which is impossible due to the margin of error.
Haphazard, hurried attempts to modernize often result in collecting data for data’s sake. Many executives unfamiliar with how AI algorithms operate will spend exorbitant amounts of labor and capital collecting data, yet set prices manually, at quarterly meetings. “Data is the new oil” doesn’t hold as much weight. Rather, the algorithm is the new oil, and algorithms can run on a very small amount of data. Facebook, for example, doesn’t need the cookies and tracking that it needed five years ago; its AI models are now sophisticated enough to guide ad placement without invading a user’s privacy.
By overhauling linear pricing models, large chain pharmacies can take the first step towards gaining an in-depth, holistic view of their customers. Sales do not exist in a vacuum. A purchase results from the culmination of time, place, seasonality, sales personnel, advertising, loyalty programs and many other intangibles. The human brain does not have the capacity to account for all these factors, but luckily AI does.
These advanced algorithms will enable customers to receive personalized care — such as recommendations for supplemental over-the-counter items, remote monitoring and improved customer service. 20% of those surveyed by McKinsey claimed “personal touch” was one of two primary factors for determining pharmacy selection. These human-like qualities will give brick-and-mortar pharmacies a much-needed edge over their online competitors.
Large chain drug stores face an uphill battle, yet, equipped with the right level of data granularity, these organizations can continue to cut a path to growth, providing life-saving care well into the foreseeable future.
Fabrizio Fantini is vice president of product strategy at ToolsGroup, a supply chain planning and optimization firm. He can be reached at ffantini@toolsgroup.com.